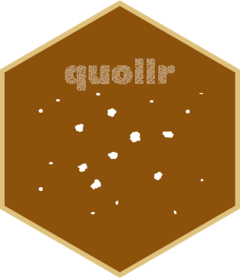
3. Algorithm for visualising the model overlaid on high-dimensional data
Source:vignettes/quollr3algo.Rmd
quollr3algo.Rmd
In here, we’ll walk through the algorithm for preprocessing 2D embedding data to construct a model overlaid with high-dimensional data.
The algorithm consists of two steps. First, construct the model in 2D space. Second, lift the model into high-dimensions. Therefore, to begin the process, first you need to know how the 2D model is constructed.
Construct the 2D model
Binning the data
To construct the model in the 2D space, first you need to hexagonally bins the 2D layout. Discussed in details in 3. Algorithm for binning data.
lim1 <- s_curve_obj$s_curve_umap_scaled_obj$lim1
lim2 <- s_curve_obj$s_curve_umap_scaled_obj$lim2
r2 <- diff(lim2)/diff(lim1)
umap_scaled <- s_curve_obj$s_curve_umap_scaled_obj$scaled_nldr
hb_obj <- hex_binning(data = umap_scaled, bin1 = 15, r2 = r2)
all_centroids_df <- hb_obj$centroids
counts_df <- hb_obj$std_cts
Obtain bin centroids
Nest step is to obtain the hexagonal bin centroid coordinates
(all_centroids_df
) and standard number of points within
each hexagon (counts_df
). Then, you can generate tibble
which gives hexagonal ID, centroid coordinates and standard counts where
data exists.
df_bin_centroids <- extract_hexbin_centroids(centroids_df = all_centroids_df,
counts_df = counts_df)
glimpse(df_bin_centroids)
#> Rows: 240
#> Columns: 6
#> $ hexID <int> 1, 2, 3, 4, 5, 6, 7, 8, 9, 10, 11, 12, 13, 14, 15, 16, 17, …
#> $ c_x <dbl> -0.10000000, -0.01736457, 0.06527086, 0.14790629, 0.2305417…
#> $ c_y <dbl> -0.08849688, -0.08849688, -0.08849688, -0.08849688, -0.0884…
#> $ bin_counts <int> NA, NA, NA, NA, NA, NA, NA, NA, NA, NA, NA, NA, NA, NA, NA,…
#> $ std_counts <dbl> NA, NA, NA, NA, NA, NA, NA, NA, NA, NA, NA, NA, NA, NA, NA,…
#> $ drop_empty <lgl> TRUE, TRUE, TRUE, TRUE, TRUE, TRUE, TRUE, TRUE, TRUE, TRUE,…
Triangulate bin centroids
Then, you need to triangulate the bin centroids.
tr1_object <- tri_bin_centroids(hex_df = df_bin_centroids, x = "c_x", y = "c_y")
str(tr1_object)
#> List of 2
#> $ trimesh_object:List of 11
#> ..$ n : int 240
#> ..$ x : num [1:240] -0.1 -0.0174 0.0653 0.1479 0.2305 ...
#> ..$ y : num [1:240] -0.0885 -0.0885 -0.0885 -0.0885 -0.0885 ...
#> ..$ nt : int 434
#> ..$ trlist: int [1:434, 1:9] 1 17 31 16 16 3 17 18 17 46 ...
#> .. ..- attr(*, "dimnames")=List of 2
#> .. .. ..$ : NULL
#> .. .. ..$ : chr [1:9] "i1" "i2" "i3" "j1" ...
#> ..$ cclist: num [1:434, 1:5] -0.0587 -0.0174 -0.1413 -0.0587 -0.0174 ...
#> .. ..- attr(*, "dimnames")=List of 2
#> .. .. ..$ : NULL
#> .. .. ..$ : chr [1:5] "x" "y" "r" "area" ...
#> ..$ nchull: int 44
#> ..$ chull : int [1:44] 1 2 3 4 5 6 7 8 9 10 ...
#> ..$ narcs : int 673
#> ..$ arcs : int [1:673, 1:2] 2 16 1 2 17 16 31 32 16 17 ...
#> .. ..- attr(*, "dimnames")=List of 2
#> .. .. ..$ : NULL
#> .. .. ..$ : chr [1:2] "from" "to"
#> ..$ call : language tri.mesh(x = hex_df[[rlang::as_string(rlang::sym(x))]], y = hex_df[[rlang::as_string(rlang::sym(y))]])
#> ..- attr(*, "class")= chr "triSht"
#> $ bin_counts : num [1:240] NA NA NA NA NA NA NA NA NA NA ...
To visualize the results, simply use geom_trimesh()
and
provide the hexagonal bin centroid coordinates. This will display the
triangular mesh for you to examine.
trimesh <- ggplot(df_bin_centroids, aes(x = c_x, y = c_y)) +
geom_trimesh() +
coord_equal() +
xlab(expression(C[x]^{(2)})) + ylab(expression(C[y]^{(2)})) +
theme(axis.text = element_text(size = 5),
axis.title = element_text(size = 7))
trimesh
Create the wireframe in 2D
To build the wireframe in 2D, you’ll need to identify which vertices
are connected. You can obtain this by passing the triangular object to
the gen_edges
function, which will provide information on
the existing edges and the connected vertices.
tr_from_to_df <- gen_edges(tri_object = tr1_object, threshold = 0)
glimpse(tr_from_to_df)
#> Rows: 313
#> Columns: 6
#> $ from <int> 1, 4, 4, 12, 2, 12, 3, 13, 13, 5, 5, 22, 32, 22, 7, 43, 23, 23,…
#> $ to <int> 2, 5, 13, 13, 3, 22, 5, 23, 14, 14, 6, 33, 44, 23, 15, 44, 34, …
#> $ x_from <dbl> 0.10658857, 0.14790629, 0.14790629, 0.10658857, 0.18922400, 0.1…
#> $ y_from <dbl> -0.01693250, 0.05463188, 0.05463188, 0.12619626, -0.01693250, 0…
#> $ x_to <dbl> 0.1892240, 0.2305417, 0.1892240, 0.1892240, 0.2718594, 0.147906…
#> $ y_to <dbl> -0.01693250, 0.05463188, 0.12619626, 0.12619626, -0.01693250, 0…
To visualize the results, you can use vis_rmlg_mesh()
.
This function enable you to observe the wireframe in 2D obtained from
the algorithm’s computations.
trimesh_removed <- vis_rmlg_mesh(tr_coord_df = tr_from_to_df) +
xlab(expression(C[x]^{(2)})) + ylab(expression(C[y]^{(2)})) +
theme(axis.text = element_text(size = 5),
axis.title = element_text(size = 7))
trimesh_removed
Lift the model into high-dimensions
To lift the constructed model into high-dimensions, you need to map the 2D hexagonal bin centroids to high-dimensions. To do that, first, you need to obtain the data set which have the 2D embedding with their corresponding hexagonal bin IDs.
umap_data_with_hb_id <- s_curve_obj$s_curve_umap_hb_obj$data_hb_id
glimpse(umap_data_with_hb_id)
#> Rows: 3,750
#> Columns: 4
#> $ emb1 <dbl> 0.27026890, 0.78809832, 0.77114044, 0.30624533, 0.54928439, 0.16…
#> $ emb2 <dbl> 0.83904768, 0.46559830, 0.31878857, 0.54166604, 0.80570434, 0.25…
#> $ ID <int> 1, 2, 3, 5, 6, 7, 9, 10, 11, 12, 15, 16, 18, 19, 24, 25, 26, 27,…
#> $ hb_id <int> 200, 132, 102, 140, 189, 79, 160, 101, 132, 35, 170, 95, 216, 17…
Next, you need to create a data set with the high-dimensional data and the 2D embedding with hexagonal bin IDs.
df_all <- dplyr::bind_cols(s_curve_noise_training |> dplyr::select(-ID), umap_data_with_hb_id)
glimpse(df_all)
#> Rows: 3,750
#> Columns: 11
#> $ x1 <dbl> -0.11970232, -0.04921160, -0.77446658, -0.47814517, 0.81769684, …
#> $ x2 <dbl> 1.6378934, 1.5091702, 1.3025775, 0.0176821, 0.9269894, 1.4012232…
#> $ x3 <dbl> -1.9928098283, 0.0012116250, 0.3673851752, -1.8782808189, -1.575…
#> $ x4 <dbl> 0.0104235802, -0.0177487701, -0.0017319658, 0.0084845242, -0.003…
#> $ x5 <dbl> 1.247143e-02, 7.263505e-03, 1.558974e-02, 5.331790e-03, -9.79905…
#> $ x6 <dbl> 0.092310860, -0.036199525, -0.096239517, 0.099753067, 0.09891648…
#> $ x7 <dbl> -0.0012762884, -0.0053483078, 0.0033535915, 0.0006769539, 0.0069…
#> $ emb1 <dbl> 0.27026890, 0.78809832, 0.77114044, 0.30624533, 0.54928439, 0.16…
#> $ emb2 <dbl> 0.83904768, 0.46559830, 0.31878857, 0.54166604, 0.80570434, 0.25…
#> $ ID <int> 1, 2, 3, 5, 6, 7, 9, 10, 11, 12, 15, 16, 18, 19, 24, 25, 26, 27,…
#> $ hb_id <int> 200, 132, 102, 140, 189, 79, 160, 101, 132, 35, 170, 95, 216, 17…
Then, use avg_highd_data()
to obtain the
high-dimensional coordinates of the model.
df_bin <- avg_highd_data(data = df_all)
glimpse(df_bin)
#> Rows: 125
#> Columns: 8
#> $ hb_id <int> 18, 19, 20, 34, 35, 36, 37, 38, 39, 40, 41, 48, 49, 50, 51, 52, …
#> $ x1 <dbl> 0.99930247, 0.94287531, 0.70062586, 0.96707217, 0.81517204, 0.51…
#> $ x2 <dbl> 0.06589512, 0.09959561, 0.05541615, 0.35401749, 0.31058901, 0.26…
#> $ x3 <dbl> 1.0309217, 1.3117090, 1.7012223, 1.2250527, 1.5684607, 1.8482762…
#> $ x4 <dbl> -0.0033356106, 0.0066771925, 0.0012777401, -0.0011772351, -0.000…
#> $ x5 <dbl> 1.224449e-03, -2.129098e-03, -6.202391e-04, 1.234268e-03, -3.318…
#> $ x6 <dbl> 0.015545625, 0.033324342, -0.014773297, -0.001446298, -0.0049625…
#> $ x7 <dbl> -1.373899e-03, -1.270457e-03, -2.645191e-03, -6.119639e-04, 1.79…
Result
Finally, to visualise the model overlaid with the high-dimensional
data, you initially need to pass the data set with the high-dimensional
data and the 2D embedding with hexagonal bin IDs (df_all
),
high-dimensional mapping of hexagonal bin centroids
(df_bin
), 2D hexagonal bin coordinates
(df_bin_centroids
), and wireframe data
(distance_df
).
df_bin_centroids <- df_bin_centroids |>
dplyr::filter(std_counts > 0)
df_exe <- comb_data_model(
highd_data = s_curve_noise_training,
model_highd = df_bin,
model_2d = df_bin_centroids)
tour1 <- show_langevitour(point_df = df_exe, edge_df = tr_from_to_df)
tour1